How does Netflix manage to show you a movie without interruptions?
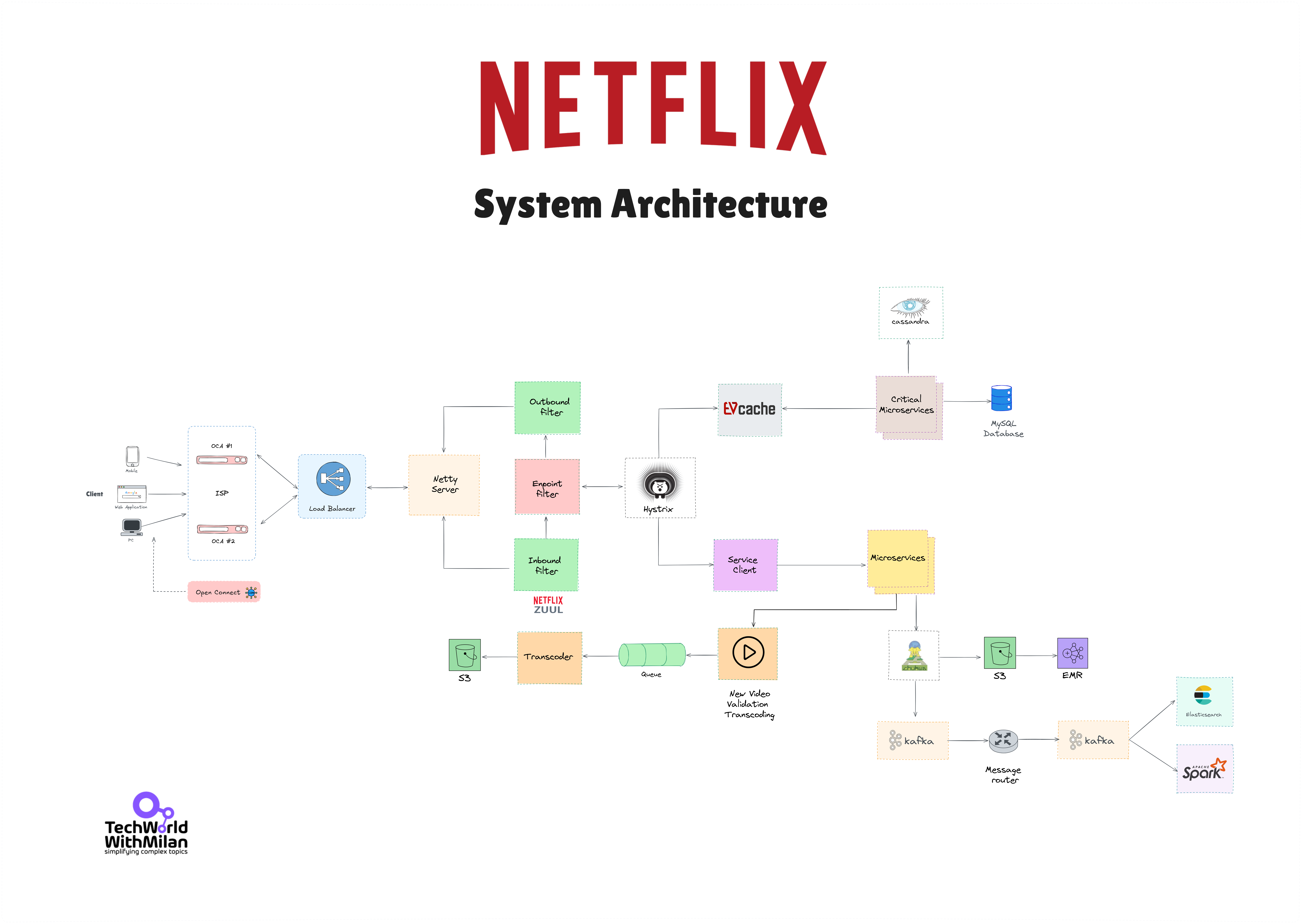
-
Netflix's recommendation system leverages advanced machine learning and data analytics to personalize content for users. It analyzes viewing history, ratings, and behavioral patterns to predict preferences, using a combination of collaborative filtering, deep learning, and A/B testing to refine suggestions. The system balances global trends with individual tastes to maximize engagement.
-
Core Technical Concepts/Technologies:
- Collaborative filtering (user-user and item-item)
- Deep learning models (neural networks for feature extraction)
- A/B testing frameworks
- Real-time data processing (Apache Kafka, Flink)
- Distributed storage (Cassandra, S3)
- Microservices architecture
-
Main Points:
- Personalization Engine: Combines explicit (ratings) and implicit (watch time, pauses) signals to train models.
- Algorithm Diversity: Uses hybrid approaches (collaborative + content-based filtering) to avoid "filter bubbles."
- Scalability: Handles 250M+ users via distributed systems (e.g., microservices for recommendations, Cassandra for metadata).
- Real-Time Updates: Processes interactions (e.g., skips, rewinds) in near real-time using Kafka/Flink pipelines.
- Experimentation: Runs thousands of A/B tests yearly to optimize UI, thumbnails, and ranking algorithms.
-
Technical Specifications/Examples:
- Model Training: TensorFlow/PyTorch for deep learning models; embeddings represent users/items in latent space.
- Code Snippet (Pseudocode):
def recommend(user_id): user_embedding = model.get_embedding(user_id) similar_items = item_embeddings.cosine_similarity(user_embedding) return rank(similar_items)
- Infrastructure: AWS EC2 for compute, S3 for storage, and Titan for feature management.
-
Key Takeaways:
- Hybrid algorithms (collaborative + content-based) improve recommendation diversity.
- Real-time feedback loops are critical for accuracy.
- Scalability requires decoupled microservices and distributed databases.
- Continuous A/B testing drives incremental improvements.
-
Limitations/Caveats:
- Cold-start problem for new users/items remains challenging.
- Bias mitigation (e.g., over-recommending popular content) is an active research area.
- Trade-offs between personalization and serendipity (explore-exploit dilemma).
A deep dive in the Netflix systems architecture.
This article was originally published on Tech World With Milan
Visit Original Source